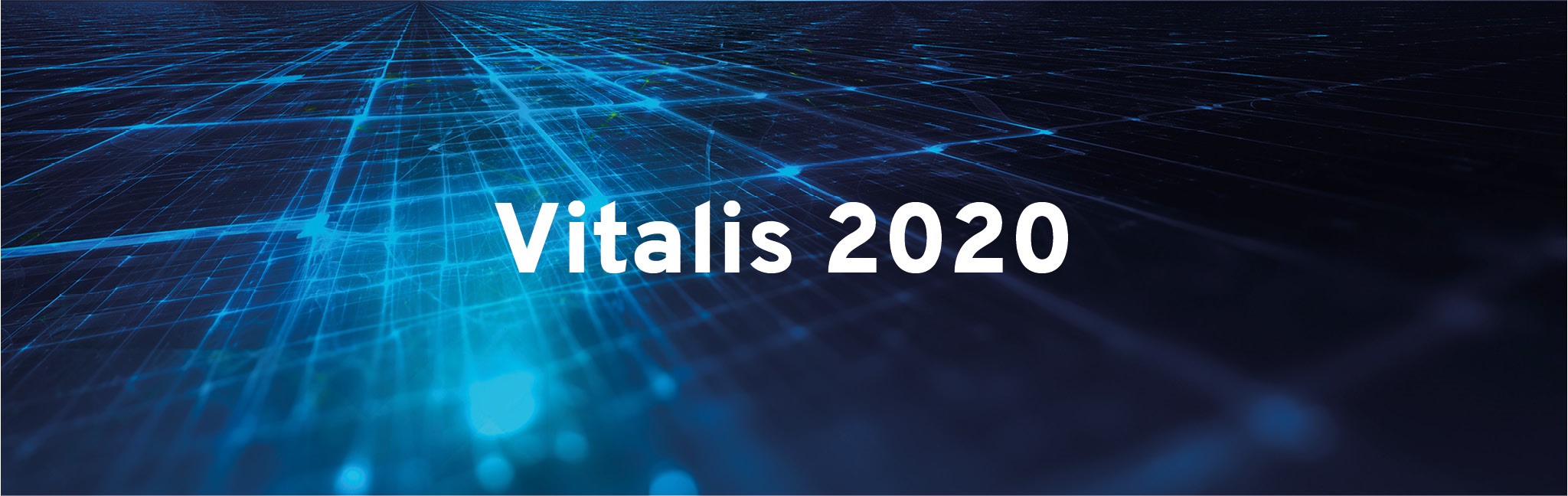
Optimised Real Time Demand/Capacity Management at Danish hospitals with Machine Learning based Forecasting – Results from a research project Har passerat
Onsdag 26 augusti 2020 13:30 - 14:00 Kliniska beslutstöd
Föreläsare: Lene Buch
A Big Data research project called DABAI (2016-2020) aims to discover if machine learning can improve the execution of real time demand/capacity management used in all Danish acute hospitals. The processes are put in place to coordinate admission management, discharge management, overflow situations and cross hospital patient flow in general. The project participants include researchers from Aarhus University Computer Science and 8 hospitals in Denmark. The motivation behind the project was firstly, the fact that hospitals get fewer and fewer beds for patients. This calls for an optimised patient flow to ensure that the right patient is in the right bed, at the right time – and only as long as it is necessary from a clinical perspective. Secondly, it can be challenging to ensure right placing of acute incoming patients in the hospital at admission. Furthermore, if the patient has to be transferred from one ward to another, it presents a risk of lost information and a potential prolonging of the patient’s admission to the hospital. Thirdly, every time a patient is discharged, an evaluation is made based on the patient’s physical and psychological wellbeing, test results, severity of current illness, comorbidity, social situation and ability to self-care after discharge. All this information and clinical data can be used for forecasting the risk of readmission. Following this, the three focus areas of the project are: (1) Making a forecast of patients flow into each department per hour and per day; (2) forecasting the “Length of stay” per patient and (3) forecasting the “Risk of readmission” per patient. It was the aim to build at tool that provide support to the clinicians in placing the patients at the right ward – emergency, acute ward or bed ward by presenting the forecast of the patient’s length of stay at the admission to the hospital. And support the clinicians in discharging the right patients at the right time based on the forecast of risk of readmission and visualizing in which clinical areas the risk was e.g. high value of bloodtest, high clinical values e.g. temperature, result of a x-ray combined with diagnoses and more. The project tests and evaluates in the 8 hospitals, whether machine learning based forecasting can support the clinicians in making the right discharge decisions. The forecast can oversee a lot of data and present forecasts to the clinicians and use explainable AI. The forecasts will be ready and implemented in the winter 2019-2020 and the hope is, that the forecasts will be so accurate that the clinicians will use the forecasts as reliable decision support in their daily practice. In this workflow they decide in which ward, they will admit the patient, to make the best Patient Flow possible – and take the forecast into account, when they decide when a patient can be discharged with the lowest risk of readmission. The results of the research project will be revealed at this presentation.
Språk
Engelska
Ämne
Beslutstöd
Föreläsningssyfte
Övrigt
Nivå
Fördjupning
Målgrupp
Chef/Beslutsfattare
Tekniker/IT/Utvecklare
Forskare (även studerande)
Omsorgspersonal
Vårdpersonal
Nyckelord
Exempel från verkligheten
Nytta/effekt
Personcentrering
samverkan över organisationsgränser
Innovativ/forskning
Test/validering
Appar
Patientsäkerhet
Användbarhet
Seminarietyp
Inspelad föreläsning
Föreläsare
Lene Buch Föreläsare
Senior Clinical Adviser
Systematic A/S
Lene Buch is a Senior Clinical Adviser at Systematic with a clinical background and a Masters degree in Healtcare Informatics. She has worked with health IT for many years. Her primary focus is on innovation and development of health IT in collaboration with clinicians.